Progressing HR with Dave Millner
30.07.2020
About the series:
"Progressing HR" is a weekly series on our blog featuring the views of experts on the digitalisation of HR with regards to bias and recruitment.
About the author:
Dave Millner, also known as the HR Curator, is an HR futurist and a regular presenter at conferences
promoting the future of HR, the role of technology and the need for analytics. He is
an associate with Corporate Research Forum (CRF), Hult Ashridge Business School
and the Centre for Effective Organizations at University of Southern California
promoting the future of HR, the role of technology and the need for analytics. He is
an associate with Corporate Research Forum (CRF), Hult Ashridge Business School
and the Centre for Effective Organizations at University of Southern California
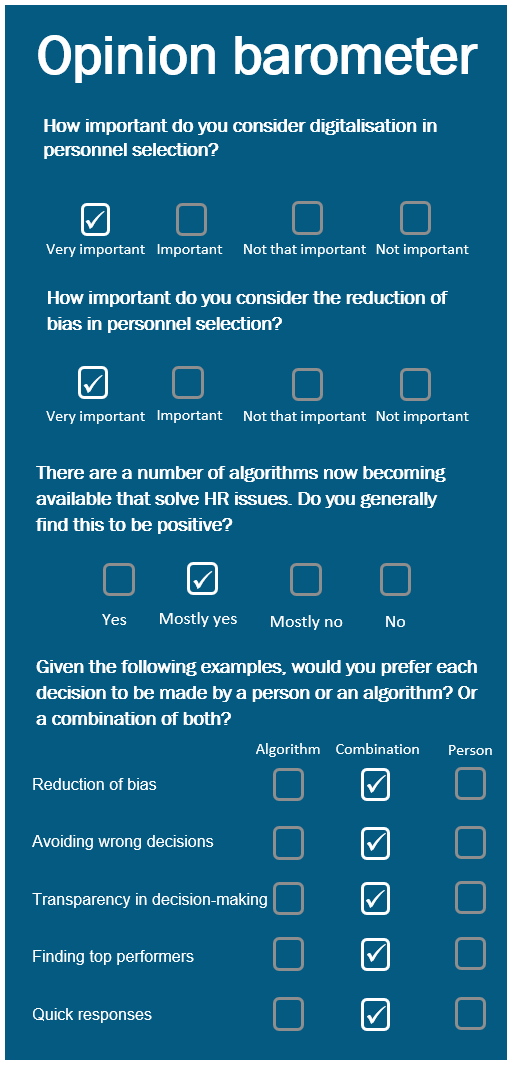
Do you see potential for the use of algorithms in personnel selection? Where are the risks?
Recruiting-based algorithms, from a range of studies and case studies, have highlighted that this type of evidence based approach can drive and support the attainment of higher quality hires. There is undoubted potential in the approach as it can raise objectivity in candidate assessment, help to reduce bias and identify applicants that are more likely to become productive employees who want to stay in a job and in your organisation for longer periods of time.
However with increasing automation, such as Artificial Intelligence (AI) and Machine Learning (ML) based applications, it is easy to accept that the data and the algorithms that they provide are correct. From my perspective there are some key questions that need to be asked with such applications:
• What is the algorithm actually measuring?
• What population samples were used to build the algorithm?
• What evidence exists to suggest that it is a good algorithm?
• What are the variables used by the algorithm?
• What are the variables used by the algorithm?
• How is the algorithm assessing or scoring employees?
• What steps have been taken to remove adverse impact? This is about what steps have been taken to ensure that data is both processed fairly and that it represents the target population.
• What feedback or outcomes can candidates expect to receive from the process?
Automation of the recruitment process requires careful ethical judgements to be taken so never under-estimate the need to question and challenge vendors about their products and solutions. Ensure you consider these practical elements when shifting to more of a technology-based approach:
• Will your prospective candidates have connectivity? Everyone involved in virtual assessment processes will need to be using a strong and reliable internet connection.
• Are your competencies and criteria for jobs future proofed? There is no point using a job description from years ago as you need to be describing the future and the role within it.
• How are you intending to monitor for bias? What training and supervisory processes are in place to ensure that best practices are maintained.
• How do you intend to maintain the high levels of candidate experience and care? Virtual is about efficiency and focus based upon data production but we must never lose the human touch.
• How will the recruitment data used in the decision-making process be utilised in onboarding, performance management and learning & development decisions once the employee has joined?
• Is the virtual process as thorough as the in-person process? Maintaining equivalence of the process and the rigour of decision making is an important consideration when moving to a virtual environment.
• Are you able to offer candidate feedback for all? Automating candidate feedback via technology will ensure that candidates get something back in return for their time and effort.
You might think that answering these types of questions is both complex and ‘over the top’; the issue is that without knowing the answers to these type of challenges will mean that you are instigating an automated process ‘blind’ and that is a business risk that organisations cannot afford to take.
To what extent do you think bias plays a role in personnel selection?
As humans, we are hardwired to make decisions and the way we think is largely shaped by various unconscious biases which ultimately influence the way we perceive the world around us. These could include how we were brought up, how we were socialised, our subsequent social groups, our exposure to diversity in society and the media at large are all aspects of bias that feed into our decision-making process and ultimately help us form our judgements.
Ideally the decision to hire a candidate would be based solely on their capability to do a job well. The hire should be approached in an objective, pragmatic way, free from subjectivity and unconscious bias but we don’t live in an ideal world, and therefore outside factors can cloud our judgement. These include the well known ‘halo and horns’ effect, making decisions based on perceived truths and then spend the rest of the interview to, subconsciously or not, try to justify our bias, and judging someone’s suitability for a job based on superficial factors that have no significant relevance to how they may operate in the job.
The challenge throughout all of this is that this unconscious bias happens whether we want it to or not, it’s unconscious.
Can algorithms help to reduce bias in personnel selection?
There is no doubt that algorithms can help to reduce bias in recruitment processes. However, we need to be very mindful that any algorithm is based upon data and that in reality, algorithms can often mirror the unconscious biases that already exist. Hence the questions raised earlier become very relevant to challenging the robustness of the algorithm itself; lots of complex data sets doesn’t always mean that biases do not exist.
Stress the need for better data and build continual checkpoints into your hiring pipeline. For example, there was the recent example when Amazon’s hiring software learnt that male applicants tended to have more experience than female applicants when it came to the technology sector. As a result of that the system started to filter out women’s applications as a shortcut because of that knowledge. Even smart machines can make assertions that are reliable!
Allow your tools to make your recruiting process better and your humans to make your tools better. In Amazon’s case, it was the recruiting team who noticed that resumes were overwhelmingly male and focused on why that was. Instead of assuming that the data was entirely objective, Amazon decided to stop the whole project because it wasn’t yet sufficiently robust.
Algorithms are a critical part of enabling recruitment teams to find the right person for the right job at the right time. Data provides the evidence and insights that enable them to make better informed decisions but that relies upon ensuring that the data informs rather than dictates every decision.
What do you think is the ideal process for finding the right hire?
My ideal process would revolve around the following:
• Advertise the job using a profile that revolves around performance outcomes and future based capabilities, otherwise you are recruiting for the old job and not not the job as it evolves in the future.
• Assess candidates suitability via a range of online simulation and tests. These would include testing their aptitude, logic, reasoning and personality/motivational profile.
• An algorithm would then assess their suitability for the job by matching their profiles against those of previously successful employees.
• This would be followed up by an intelligent video interview, again with algorithms assessing multiple data points against critical job criteria.
• The final part of the process would then be:
(a) an Assessment Centre with recruiters and business leaders where a final decision is made based upon the data collected if the role was complex and business critical.. (b) a criteria based interview(s) for less complex roles.
The final decision would be based upon a review of all the data obtained from the process.
The process would be driven by automation and would provide candidate feedback throughout each stage. The data would be regularly reviewed to ensure that issues of bias are identified and rectified as required.