Progressing HR with Max Blumberg
16.07.2020
About the series:
"Progressing HR" is a weekly series on our blog featuring the views of experts on the digitalisation of HR with regards to bias and recruitment.
About the author:
Dr. Max Blumberg is the founder of HR analytics consultancy Blumberg Partnership which focuses on workforce transformation through data-driven analytics. He is a research fellow at Goldsmiths, University of London and a visiting professor at University of Leeds Business School.
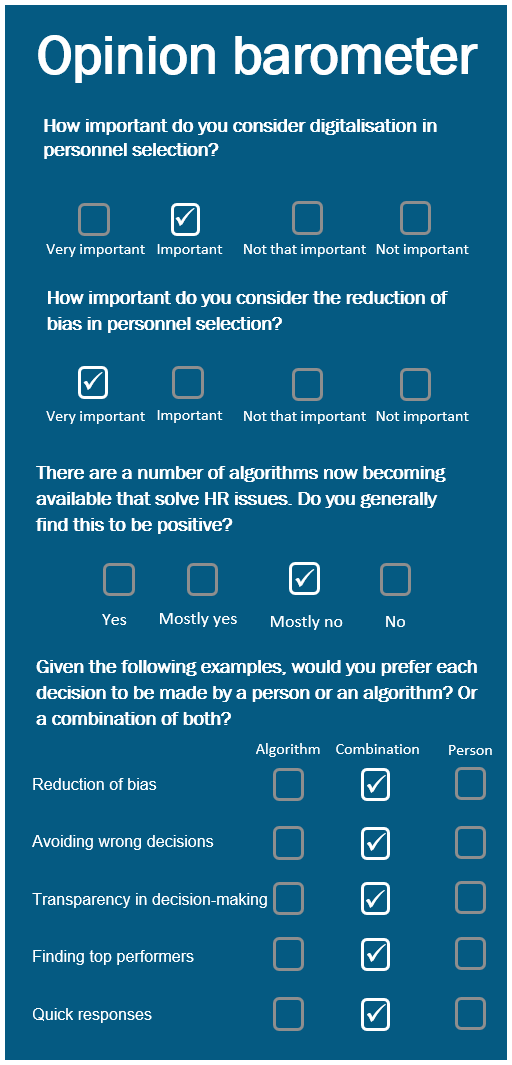
Do you see potential for the use of algorithms in personnel selection? Where are the risks?
If manual jobs shrink as a result of automation, a lot of future recruitment will focus on two generic job types which cannot be easily automated: these are roles relating to knowledge work and to “emotional labour” (such as leadership and management roles).
1. Emotional labour roles: Predicting emotions has been a Holy Grail for algorithm designers since Freud first suggested a quasi-scientific model for predicting human emotional behaviour; sadly, no algorithm has yet succeeded in predicting emotional behaviour (at least in a meaningful way).
Will algorithms ever be able to predict emotional behaviour? A best guess is that we’re probably about 25 years away from developing algorithms capable of predicting whether candidates have the “emotional” ability to manage others. This is because today’s leading-edge AI algorithms operate at the cognitive level of about a 5-year old, so it will probably take another 20 - 30 years before they reach “adulthood”.
2. Knowledge work: Algorithms are much better at selecting candidates with appropriate levels of knowledge than they are at selecting candidates capable of managing the emotions of others.
For example, algorithms are already capable of selecting effective STEM candidates; and we can expect this to extend rapidly into selecting candidates for other roles such as medicine. (Note, however, that knowledge-selection algorithms will not be able to predict the bedside manner of these health professionals’ because bedside manner entails predicting emotions - see point 1 above).
So yes, there is potential for assisting in the selection of knowledge work. The risks include the following:
1. Deploying algorithm-based selection in roles for which the algorithm is not intended (for example, attempting to select emotional competency)
2. Algorithms which are biased towards some groups more than others through inadequate "GRRACCES" calibration (GRRACCES = Gender, Race, Religion, (dis)Ability, Class, Culture, Ethnicity, Sexuality). This can lead to what psychologists and lawyers refer to as adverse impact and unsuccessful candidates can sue employers for discrimination. There is more on adverse impact below.
3. The final risk is that of developing inaccurate algorithms because they do not follow a scientific process such as the one described below
To what extent do you think bias plays a role in personnel selection?
Although we can reduce adverse impact, bias cannot be completely eliminated from algorithms or human behaviour. For example, let’s say you and I are recruiting salespeople. There are at least two interesting facets to consider when recruiting salespeople:
First, sales involve a significant degree of emotional labour (such as managing prospective customers’ emotions); thus - as per the discussion in section 1 above - we cannot use an algorithm to select candidates. Therefore, you and I will have to execute much of the selection process in person.
Second, there is not only “one type” of successful ” salesperson; successful salespeople come in a variety of different shapes, styles, cognitive attitudes, knowledge, skills and abilities; there is no single pattern. (This is true for most roles actually).
Back to you and me selecting candidates for our sales role:
In your experience as a sales manager, you may have come to believe that candidates of Type A (whatever that may be) make the best salespeople - i n contrast, my experience as a sales manager may have taught me that Type B candidates make better candidates.
The reality of this situation is that we’re both right based on our past experience - and experience is often another word for ‘bias’.
So when candidates of Type A come in, I’ll say they’re wonderful and you’ll say they’re not. And when candidates of Type B come in, our views reverse. Thus we are both selecting based on our personal bias - and in this instance, that’s OK because we could both be correct.
But where bias is not OK is when we discriminate unfairly against people based on their "GRRACCESS". So bottom-line: yes, bias plays a significant role in personnel selection, sometimes good, sometimes bad.
What do you think is the ideal process for finding the right hire?
The scientific method developed over the past 400 years and used in academia worldwide is probably the best way we know for generating valid and reliable predictions. This applies to selection as much as it applies to predicting whether a new drug is safe or not.
First identify a metric for measuring performance in the role. At worst, this metric could simply be the rating from employee performance evaluations, but we can often do better by crowd-sourcing additional performance metrics from teams within the employing company.
Now rank employees from high to low on the performance metric obtained above. If we are recruiting for future roles (e.g. for 3 years time), then we need to rank our current employees based on what we think their performance will look like in 3 years.
So now we have a list of employees in the role for which we’re recruiting ranked high to low on some company-agreed performance metric. Next, we ask managers and expert role-holders to hypothesise characteristics which might distinguish between high and low performers in the role.
Now that we know which winning characteristics predict performance, we can bake them into a recruitment algorithm which measures prospective candidates on these characteristics and then uses the statistical model developed in Step 3 to tell us the extent to which candidates look like our existing high performers.
Here is the scientific method applied to selecting employees for a given role in a particular organization:
1. Identify an outcome performance metric
2. Rank employees on the chosen performance metric
Of course the longer we project our rankings into the future, the less accurate the selection process becomes. In our experience, it is usually best to perform this process based on employees’ performance in the current year and then repeat the process annually.
3. Identify characteristics that distinguish between high and low performers
We then measure these hypothesised characteristics in our current employees, and build a statistical model to determine which of the hypothesised characteristics best predicts our performance metric (all the while remembering that correlation is not necessarily causation).
The deliverable of this step is a set of “winning” characteristics which predict performance in the role to a required level of accuracy. (There may multiple clusters of characteristics which predict high performance as discussed in the sales selection example discussed earlier).
4. Use the winning characteristics to build a selection algorithm
And there you have it: a scientific process for finding the right hire.
Can algorithms help to reduce bias in personnel selection?
Bias in personnel selection is often down to inadequate sampling when creating the algorithm. For example, if I used only UK employees when developing the scientific selection algorithm described above, there is a reasonable chance that the algorithm may be inaccurate when selecting candidates from other countries.
Algorithms can help to reduce bias in personnel selection by ensuring that representative employee samples are used when developing the algorithm.